Carnegie Mellon University has developed “Safe Construct,” a model that uses artificial intelligence to detect safety risks on construction sites.
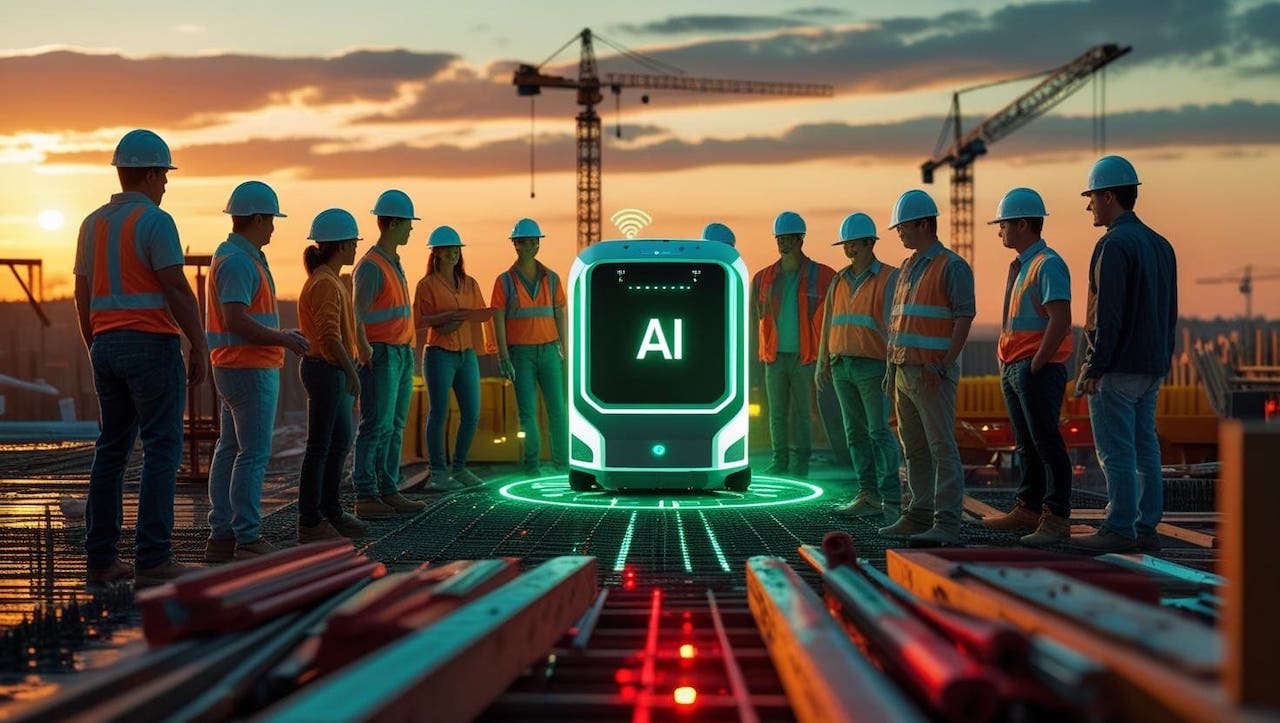
Construction sites need smarter safety tools
Workplace safety is a highly pressing issue. The rise in accidents, the difficulty in assigning responsibility, and the frequent lack of preventive measures are persistent problems. In this context, safety on construction sites plays a central role, given the inherently hazardous nature of these environments. Ensuring protection means not only improving contractor qualifications and upgrading equipment, but also adopting more effective monitoring solutions.
This is where “Safe-Construct” comes in, a new AI-powered model developed by the Department of Engineering at Carnegie Mellon University. The system uses artificial intelligence and 3D vision to enhance safety controls on construction sites.
How do you train a machine to improve safety?
The team at Carnegie Mellon took on one of the most complex and demanding applications of AI. Unlike previous efforts, which focused on clean, structured, and controlled environments, this research tackles the unpredictability of construction sites.
Their core question was: how do you train a machine to care about safety the way a human supervisor would? This goes far beyond counting hard hats or tracking worker locations. It means enabling the system to interact with real-world variables, detecting and responding to risks as they emerge.
Through the Safe-Construct project, the researchers have built a system that can interpret and react to genuine safety threats, not hypothetically, but in the field.
Teaching AI to recognize dangerous behavior
The first step was to teach the system to identify hazardous behavior, not just as visual frames or pixels, but as full-body postures and actions that may indicate a safety risk.
The major leap of this framework is moving from 2D to 3D vision.
To train the model, researchers created hundreds of simulated safety scenarios, both compliant and non-compliant. This approach helps the system learn to manage the real world’s unpredictability.
The result is a highly adaptable AI that can do more than check for a helmet. It analyzes body movements, interactions, and work procedures, offering a deeper and more contextual understanding of safety conditions. It can even detect complex violations, like whether a ladder is properly stabilized while another worker is using it, assessing multi-person tasks and tool interactions in real time.
Next step: the digital twin of the construction site
The team’s next goal is to build a digital twin of the construction site. This virtual replica would allow managers to monitor key productivity, safety, and quality metrics remotely and proactively.
The Safe-Construct model will be officially presented on June 11 at the Conference on Computer Vision and Pattern Recognition in Nashville.
The research paper, titled “Safe-Construct: Redefining Construction Safety Violation Recognition as 3D Multi-View Engagement Task”, is available on the arXiv preprint server.