For three days, Berlin hosts the tech expo connecting startups and investors in sectors like climate tech, smart cities, and data centers
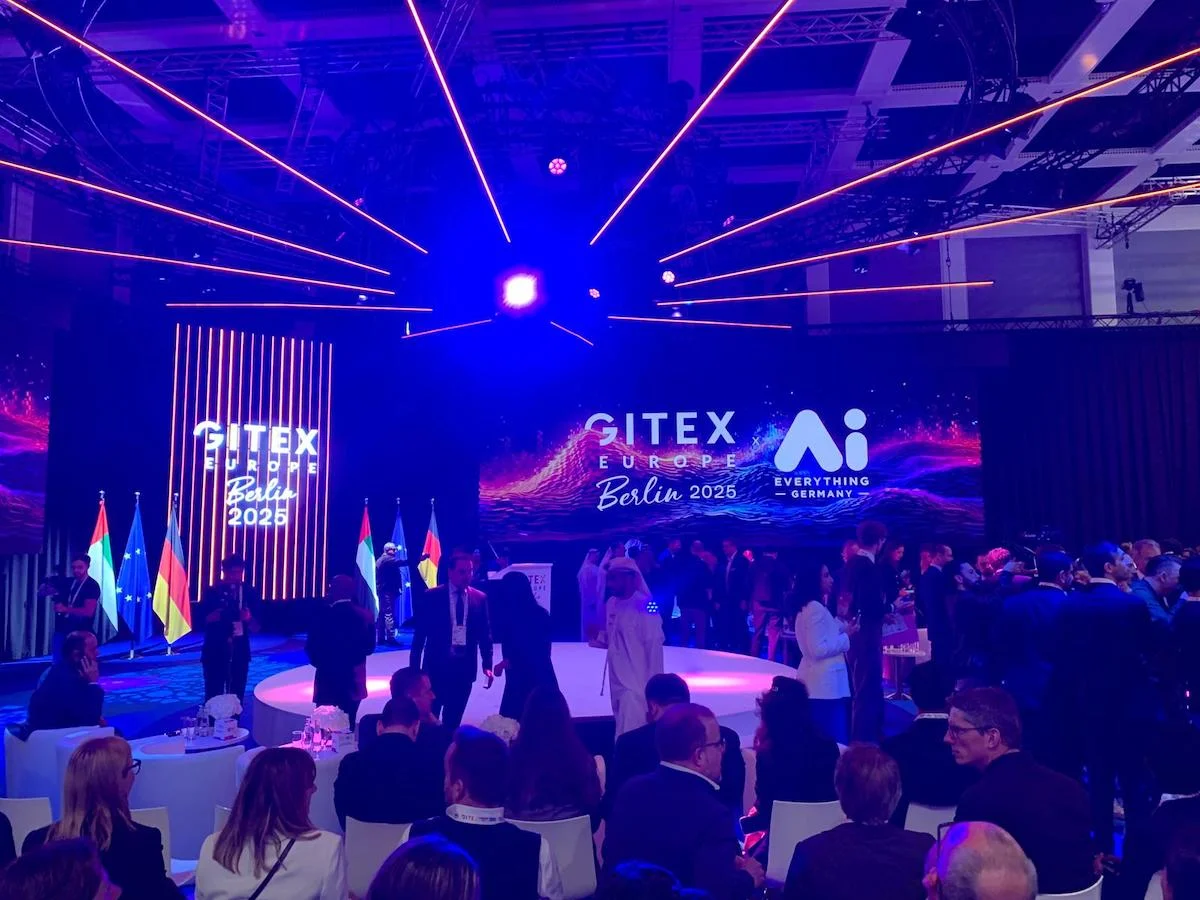
From Dubai to the heart of Europe. The Gitex tech expo, originally founded in the United Arab Emirates, has arrived in Europe for the first time, choosing Berlin as the stage to showcase how technology is reshaping our present. Gitex Europe x Ai Everything runs through May 23 at Messe Berlin.
According to the latest State of European Tech report, Europe’s tech sector will reach €2.95 trillion in 2025, five times the €518 billion it was worth just a decade ago. The startup landscape is thriving, particularly at North Star Europe, a featured event dedicated entirely to emerging ventures. A successful format in Dubai, North Star is now replicating its model in Berlin.
North Star Europe is one of the continent’s key stages for global startups, drawing over 750 companies from more than 80 countries. They connect with over 600 international investors managing €880 billion in assets. Innovation and investment are the core focus of Gitex Europe, which aims to merge an increasingly interconnected tech ecosystem: data centers, climate tech, health tech, cloud, and smart cities, all driven by artificial intelligence.
Startup innovation takes center stage
Europe’s entrepreneurial strength is amplified through a strategic partnership with the European Innovation Council (EIC), the EU’s leading deep tech accelerator. At Gitex Europe, the EIC is hosting its largest-ever startup showcase, featuring over 40 companies redefining the future of European innovation.
Among the standouts: Denmark’s Atland 3D, which produces atomic-precision nano 3D printers and collaborates with NASA, and Germany’s Kiuntra, the only global provider of cryogen-free sub-Kelvin cooling systems enabling scalable quantum infrastructure.
The unease surrounding artificial intelligence
Taking the main stage on opening day was 2024 Nobel Prize in Physics laureate Geoffrey Hinton, widely regarded as one of the founding fathers of artificial intelligence. The British scientist, who spent years working with Google, left the company in 2022 over concerns about the unchecked evolution of AI.
Still, he offered some optimism. Hinton emphasized that AI could transform medicine, pharmaceuticals, and education: “It will diagnose illnesses faster and more accurately, outperform radiologists in reading scans, and teach children and university students more effectively.” He added, “If you had a full-body MRI every year and AI interpreted the scan, nobody would need to die of cancer. It would detect nearly all tumors when they’re still very small.”
The energy dilemma of AI
So what led Hinton to step away from Google? His answer is rooted in the exponential energy demands of large-scale AI systems. “Looking five years ahead is hard. A good way to understand how hard it is, is to look five years back,” said Hinton, citing the leap in performance from large language models like ChatGPT.
“We’re reaching a point where performance gains become logarithmic. A small improvement requires doubling both data and compute power. The next increment doubles again. We’re hitting an energy ceiling,” he warned.
Why Hinton walked away from Google: power consumption
Hinton’s departure from Google was primarily driven by concerns about energy efficiency. “The brain is fundamentally analog, while these AI systems are all digital. I was working on how to build large language models using analog hardware. That would be far more energy efficient,” he said.
He explained a different approach: using voltage as neural activity and conductance as the strength of the connection. In such systems, multiplying voltage and conductance would yield charge, which neurons could sum naturally. This analog mechanism, he argues, could drastically reduce energy consumption.
What Hinton’s analog brain theory means for AI
Hinton believes the human brain operates on analog principles. Unlike digital systems that represent information in binary values (0 or 1), the brain processes information as a continuous range. Neural activity can be represented as a varying voltage, and the strength of synaptic connections as a conductance. In this model, energy efficiency is achieved by eliminating the need for constant digital-analog conversion.
Modern AI systems, including large language models, run on digital hardware. Every operation, from storing weights to processing data, relies on discrete numerical values. While this is accurate and scalable, it’s also highly energy-intensive, especially at the scale of current AI workloads.
Hinton believes the brain has a “trick” for performing complex calculations with minimal energy. That trick lies in its analog nature, and his research aims to replicate this approach in AI hardware. If successful, it could help AI systems overcome the energy barriers that threaten their sustainability.